Research
Genetic sequencing data contain a fingerprint of past evolutionary, epidemiological and ecological processes. The Computational Evolution group develops phylogenetic and phylodynamic tools in order to extract these signals from genetic sequencing data. We use our tools to address questions in a number of fields, focusing on epidemiology, public health & medicine, ecology & evolution, cell differentiation, and language evolution.
Whereas phylogenetic inference refers to reconstructing the evolutionary relationships between organisms, phylodynamic inference refers to reconstructing the dynamics that led to the observed relationships. A crucial aspect of this process is calculating the probability density of an observed phylogeny, while taking into account the possibility of unsampled and extinct lineages. This probability density is based on a model that describes the growth of the tree, usually either a birth-death or a coalescent process.
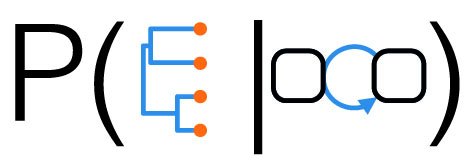
When performing inferences within a Bayesian framework, this probability density is used as a prior density for the phylogeny (the so-called tree-prior). In this way we can calculate the posterior distributions for the model parameters we want to infer, while integrating out uncertainty in other quantities. For instance, we can directly infer ecological or epidemiological parameters from genetic sequencing data while taking into account phylogenetic uncertainty.
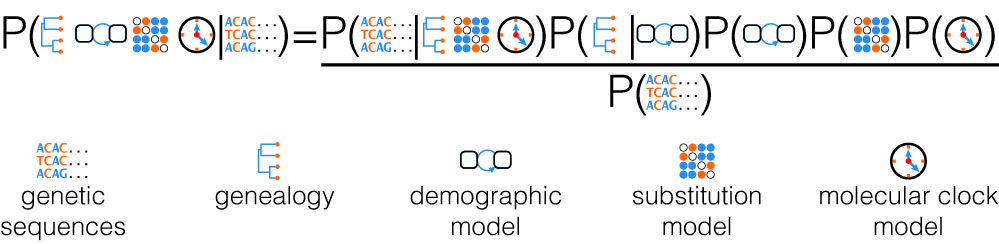
In our daily work, we define and analyze stochastic models, implement computational methods, analyze empirical data, and discuss our new insights with clinicians, public health policy makers, as well as ecologists and paleontologists.