Graph Kernels Survey
Introduction
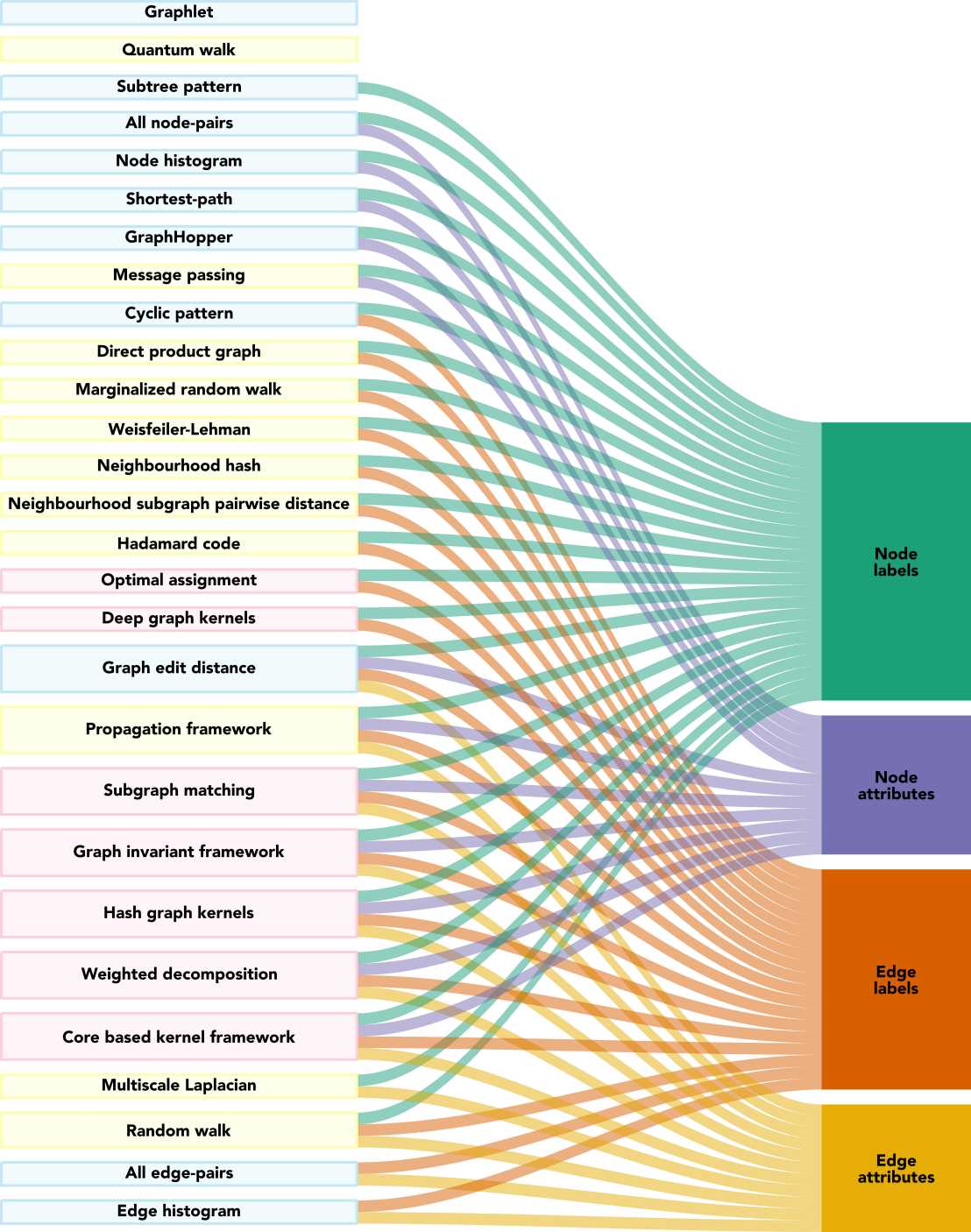
Graph-structured data are an integral part of many application domains, including chemoinformatics, computational biology, neuroimaging, and social network analysis. Over the last two decades, numerous graph kernels, i.e. kernel functions between graphs, have been proposed to solve the problem of assessing the similarity between graphs, thereby making it possible to perform predictions in both classification and regression settings. Our review covers existing graph kernels, their applications, software plus data resources, and an empirical comparison of state-of-the-art graph kernels.
Code
The code used for our experiments is available on external pageGitHubcall_made.
Dataset
Our paper used datasets from the external pageTUDortmund benchmark datasets for graphscall_made repository. The repository has since been migrated to external pagewww.graphlearning.iocall_made.
Figures
The visualizations of the graph kernels used in the paper are available as a PDF Downloadhere (PDF, 2.8 MB)vertical_align_bottom.
Contributors
Karsten Borgwardt
Elisabetta Ghisu (alumna)
Felipe Llinares-López (alumnus)
Leslie O'Bray
Bastian Rieck (alumnus)
References
Graph Kernels: State-of-the-Art and Future Challenges
Karsten Borgwardt, Elisabetta Ghisu, Felipe Llinares-López, Leslie O'Bray, and Bastian Rieck
Foundations and Trends® in Machine Learning 2020, 13 (5-6): 531-712.
external pageOnlinecall_made | Project page | external pagearXivcall_made